Research | Artificial intelligence risk management framework (AI RMF 1.0)
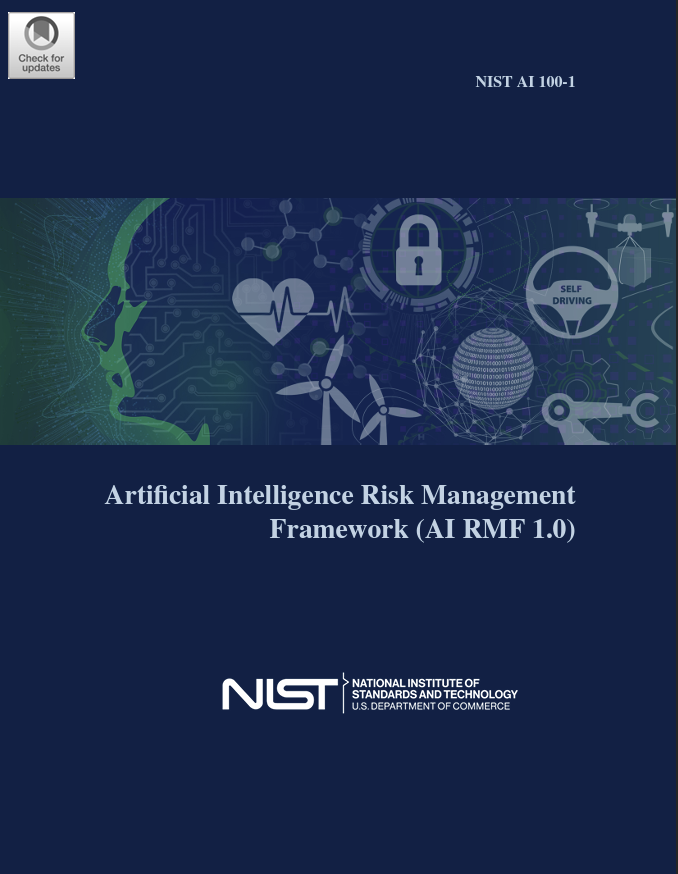
Artificial intelligence risk management framework (AI RMF 1.0)
25 January 2023
This framework is a guide to promote safe, secure and transparent use of AI systems. The Framework provides four key functions – govern, map, measure and manage – with further categories and subcategories for risk management in AI systems.
Please login or join for free to read more.