Research | Artificial intelligence solutions to support environmental, social, and governance integration in emerging markets
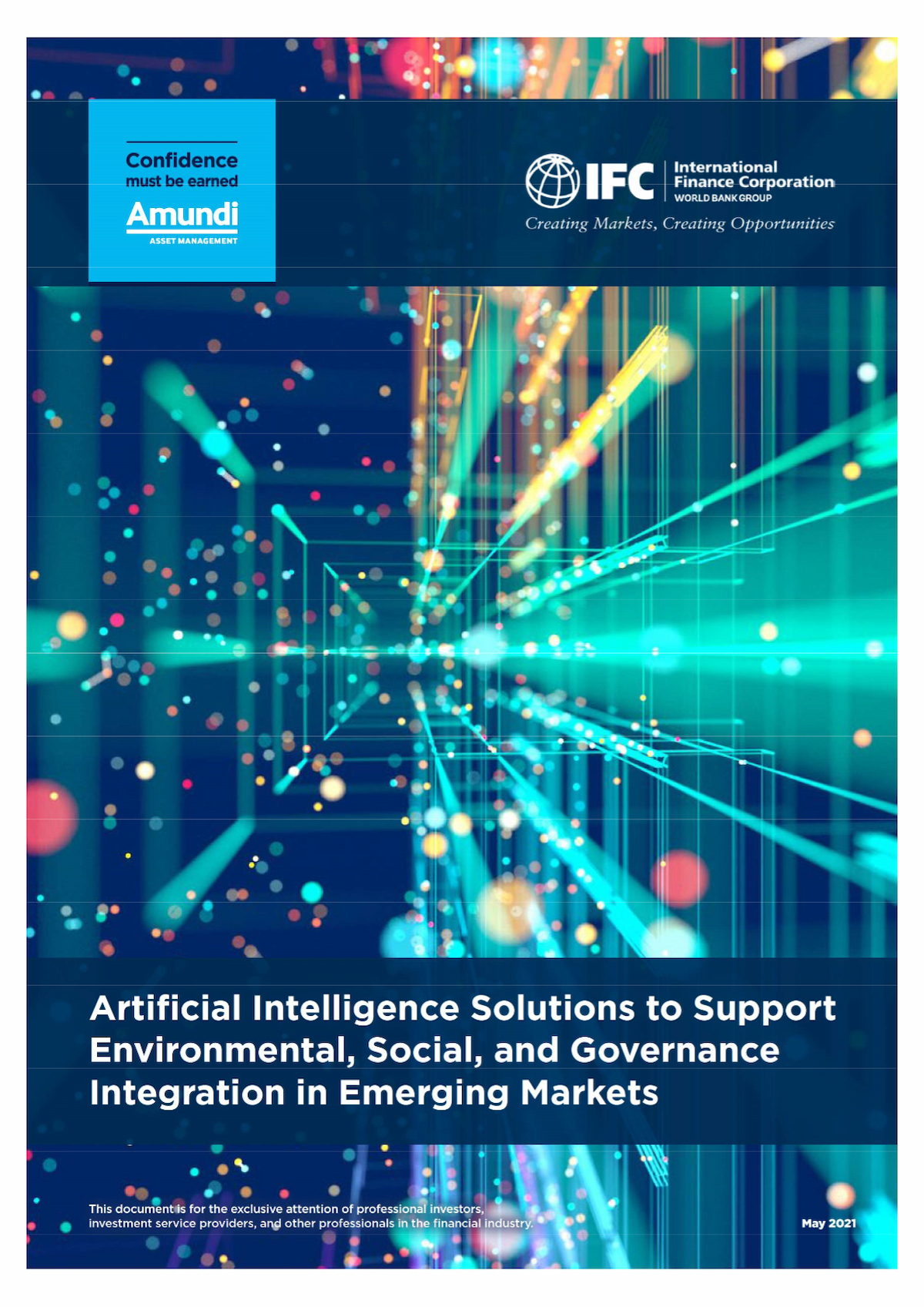
Artificial intelligence solutions to support environmental, social, and governance integration in emerging markets
31 May 2021
This report examines the use of artificial intelligence technologies to analyse environmental, social and governance (“ESG”) data for investments in emerging markets. It gives a detailed account of an experiment conducted to determine the effectiveness of such technologies in analysing the ESG performance of emerging markets issuers.
Please login or join for free to read more.