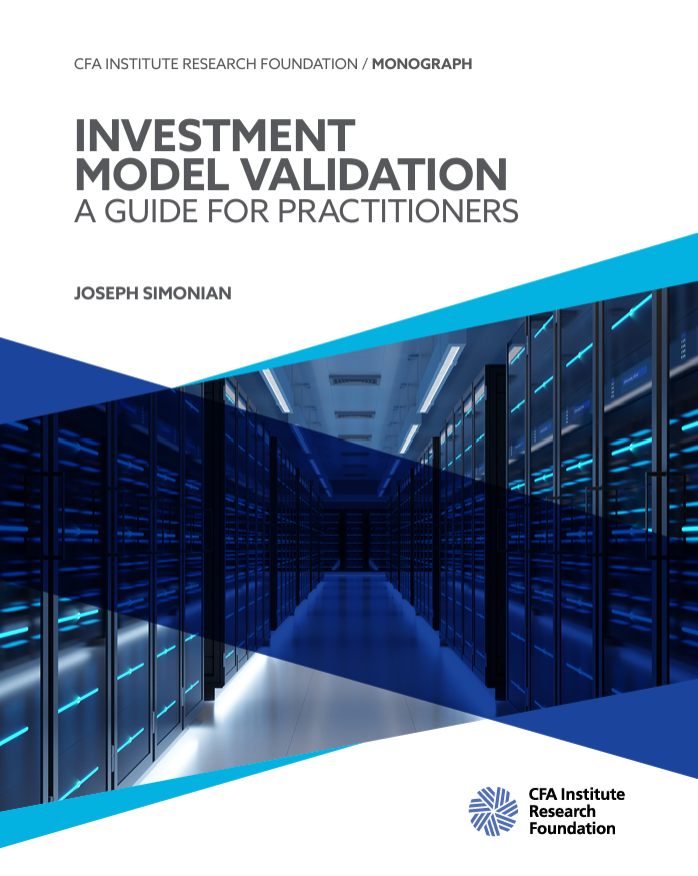
Investment model validation: A guide for practitioners
This guide offers a practical framework for assessing the robustness of investment models used in finance. This report covers methodologies such as backtesting, cross-validation, and scenario analysis to mitigate model risk, providing investment professionals with tools to enhance decision-making processes and model reliability. The guide stresses the importance of aligning model outcomes with economic theory and offers insights into performance benchmarking.
Please login or join for free to read more.
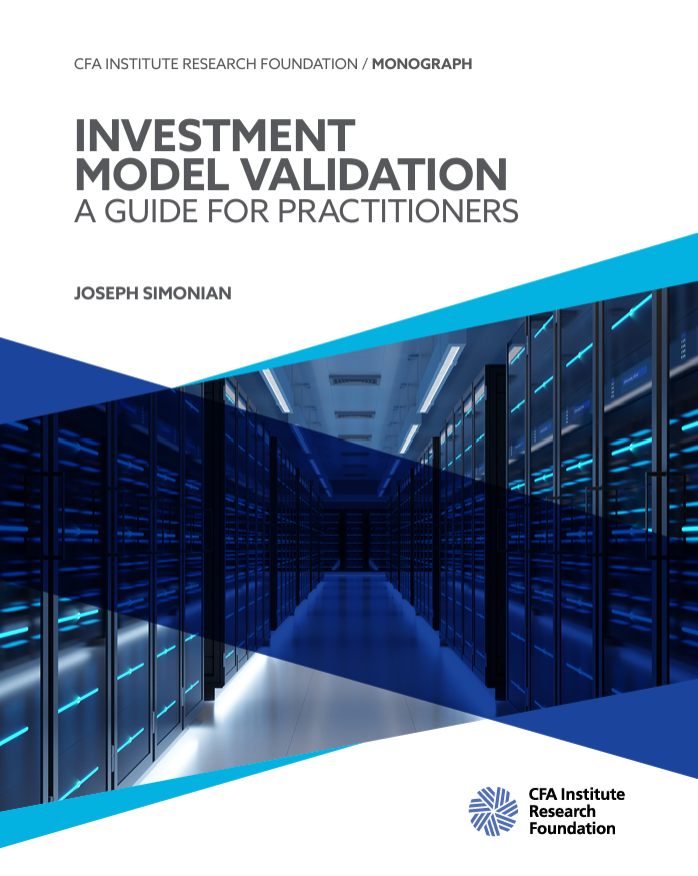
OVERVIEW
Introduction: The importance of model validation to financial product development
Model validation is critical in ensuring that financial models perform within expectations and provide reliable insights. Investment strategies rely heavily on these models, and their robustness is essential in navigating market complexities. A key challenge is the data scarcity that finance faces, making comprehensive validation processes necessary to mitigate risks and ensure reliability.
A working philosophy of model validation
The primary goal of model validation is to ensure the model aligns with business needs and market realities. One significant challenge is that financial data, unlike data in natural sciences, is sparse and cannot be manipulated under controlled experiments. The reliance on human behaviour as a market driver adds complexity to model validation, as it introduces psychological and intentional elements that are hard to predict.
Backtesting
Backtesting involves running a model against historical data to evaluate its potential performance. This process helps researchers identify the strengths and weaknesses of their models. For example, backtesting provides insights into how a strategy may react under different market conditions or during various economic regimes. However, backtesting must be approached with caution. A key limitation is that past data may not reflect future conditions, which could render models ineffective in real-world applications.
One of the main pitfalls of backtesting is overfitting—where models perform well historically but fail under new conditions. Additionally, transaction costs, market liquidity, and trade sizes can impact model performance. Recommendations suggest continual backtesting and refining models as market conditions evolve to ensure models remain adaptable and relevant.
Cross-validation
Cross-validation serves as an extension to backtesting by introducing out-of-sample testing, which helps mitigate the risk of overfitting. Techniques such as k-fold cross-validation and time-series cross-validation are used to assess how models generalise across different datasets. These methodologies help in evaluating a model’s robustness and reliability.
The report highlights that while cross-validation helps address issues in traditional backtesting, there is still a risk of overfitting, particularly in time-series data. The concept of block time-series cross-validation has been introduced as a solution to reduce memory effects in time-dependent datasets.
Performance measurement and benchmarking
Benchmarking provides context for evaluating a model’s performance by comparing it to a reference point. Effective benchmarking requires the model to be measurable, investable, and appropriate for the investment style. For instance, fixed-income benchmarks often present challenges due to the complexity of bond risk exposures. The report emphasises that a strong benchmark is crucial for validating models, as it ensures consistency in performance evaluation.
Additionally, performance metrics such as the information ratio (IR) and the Treynor ratio are key in understanding how much value a model adds relative to its risk. These metrics enable investment professionals to measure both absolute and relative returns, helping them improve their strategies.
Simulating alternative histories with synthetic datasets
The report introduces the use of Monte Carlo simulations, bootstrapping, and newer techniques like generative adversarial networks to generate synthetic datasets. These methodologies help in overcoming the limitation of data scarcity by creating hypothetical scenarios to test models. The use of these simulations adds an additional layer of robustness to model validation.
Stress testing and scenario analysis
Stress testing allows investment professionals to evaluate how models respond to extreme market conditions, such as economic shocks or political turmoil. Scenario analysis further helps in understanding a model’s resilience by assessing its behaviour under multiple hypothetical situations. The report underscores the importance of these tools in validating models, ensuring they are prepared for real-world challenges.
Conclusion
Investment model validation is a dynamic and essential process for financial professionals. It combines empirical testing, cross-validation, and performance benchmarking to provide a holistic assessment of model reliability. Through the methodologies outlined in this guide, practitioners can enhance their model validation processes, improving decision-making and mitigating risks in their strategies.