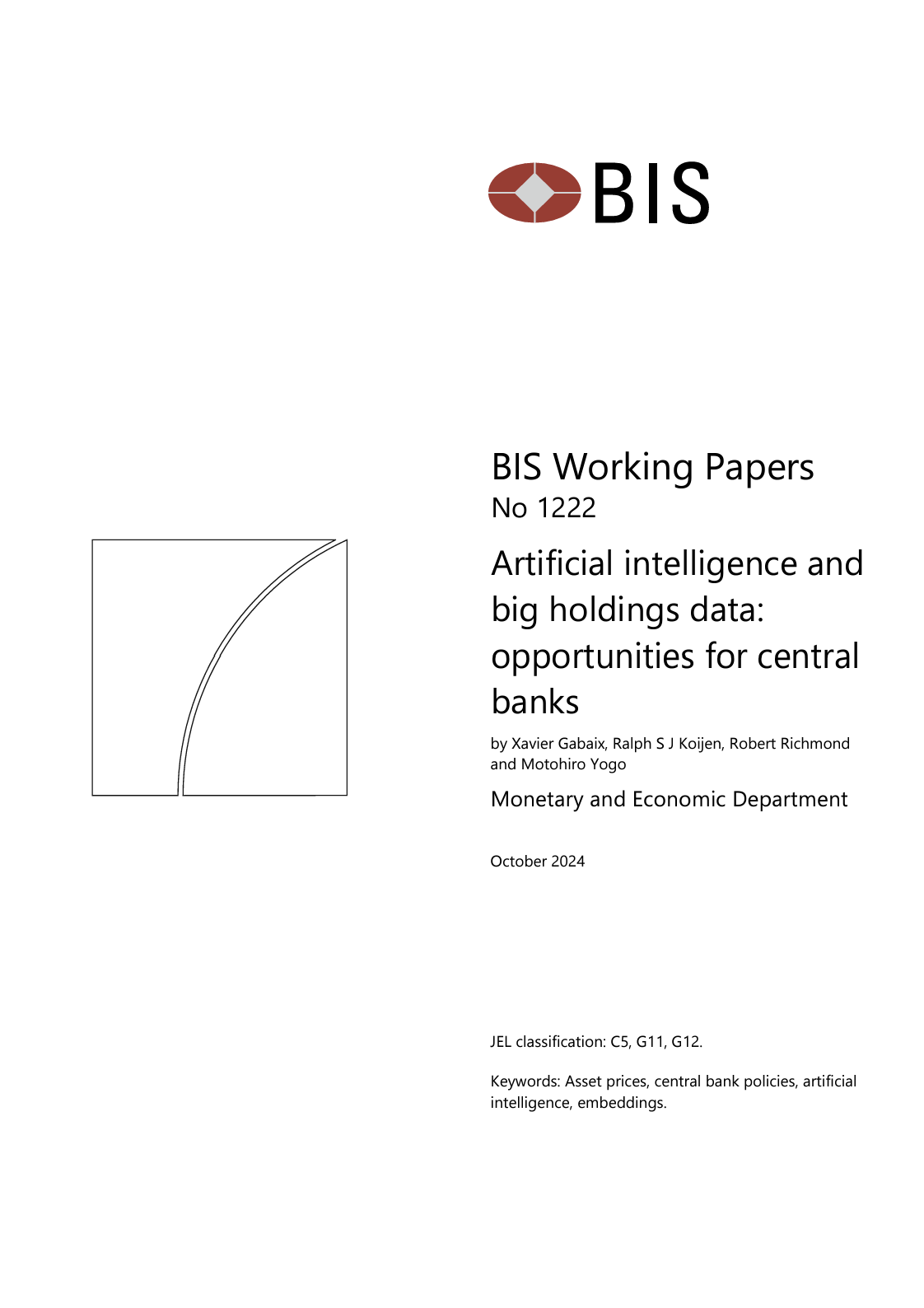
Artificial intelligence and big holdings data: Opportunities for central banks
This report explores the potential of artificial intelligence and big holdings data for central banks. It highlights how asset demand systems and AI models improve policy decisions, optimise monetary interventions, and address financial risks. Applications include managing contagion, designing climate stress tests, and identifying crowded trades, enhancing economic resilience.
Please login or join for free to read more.
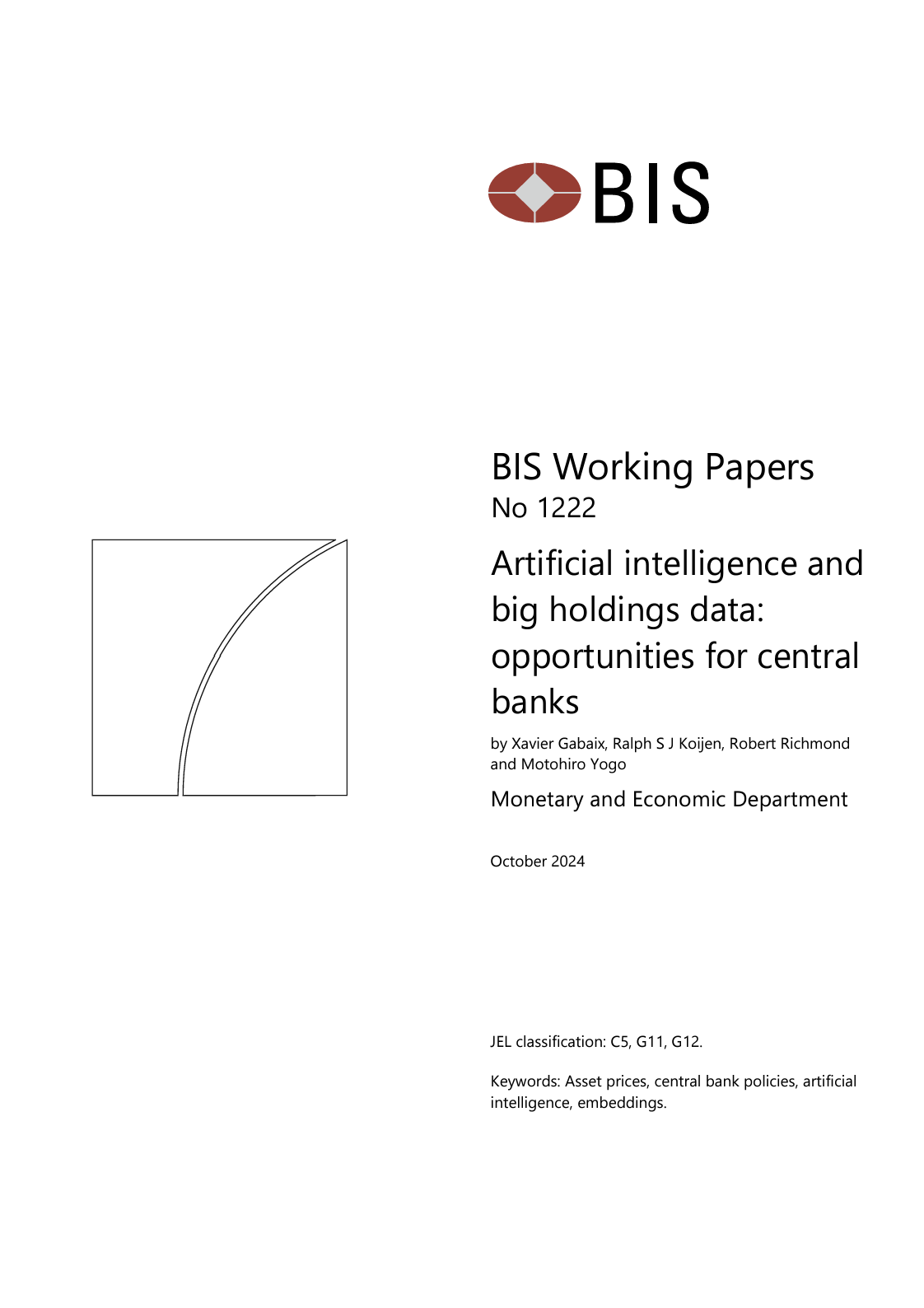
OVERVIEW
Introduction
This report investigates how artificial intelligence (AI) and big holdings data can enhance the operational and policy capabilities of central banks. It emphasises the role of AI-driven asset demand systems in understanding investor behaviour, improving financial stability, and crafting optimal policies. A key takeaway is the application of advanced embedding techniques from AI to improve the modelling of asset demand systems, which can refine predictions and decision-making processes.
A demand system approach to central banking
Improved information for policy decisions
The report outlines how demand systems can trace changes in asset prices to shifts in investor demand or supply changes. This methodology is vital for understanding financial contagion, as demonstrated during the 2009-2010 European sovereign debt crisis. For example, latent demand played a significant role in spreading contagion to Italy and Portugal, where European investors drove 96% and 64% of yield volatility, respectively.
Demand systems also offer insights into key asset prices relevant to central banks, including exchange rates, equity prices, and bond yields. These systems allow policymakers to understand fluctuations in financial condition indices like the ECB’s Composite Indicator of Systemic Stress. Notably, household investors contributed 46.9% to stock return variance during the 2008 financial crisis, highlighting their significant market impact compared to institutions.
Designing optimal policies
Central banks can use demand systems to evaluate unconventional monetary policies, such as asset purchase programs. For example, foreign investors exhibited high price elasticity during ECB interventions, amplifying the program’s effects. Moreover, demand systems can guide currency reserve management by quantifying their impact on exchange rates and convenience yields. A 2020 analysis revealed foreign exchange reserves explained 10% of exchange rate movements and contributed 0.94 percentage points to the dollar’s convenience yield.
Climate stress tests also benefit from demand systems, assessing how regulatory policies might steer investments from “brown” to “green” assets. Such frameworks enable policymakers to predict the financial market implications of environmental risk regulations.
Using AI to improve models of the asset demand system
Embeddings to measure similarity between assets and investors
AI techniques, such as embeddings, enable improved modelling of asset and investor similarities. These embeddings, derived from portfolio holdings data, allow central banks to predict investor behaviour and substitution patterns more accurately. For instance, clustering firms by embedding similarity can identify shared risks and growth opportunities. Using earnings call data, a retrieval-augmented generative model offers narrative explanations of asset clusters.
Applications of embeddings to central banks and financial stability regulators
Embeddings enhance understanding of monetary policy channels by quantifying asset substitution during asset purchase programs. They also help identify crowded trades, a common source of market instability. For example, during the 2023 AI stock surge, embeddings identified shifts from hardware to utilities stocks as market dynamics evolved. Additionally, embeddings support stress test design by predicting investor trades and their market impacts under various scenarios.
Economic applications of embeddings beyond financial markets
Beyond finance, embeddings have applications in modelling inflation and relative price dynamics. By analysing consumer purchasing patterns, central banks can track inflation trends and understand the drivers of price changes across different goods and services.
Further discussion
AI’s rapid evolution presents opportunities and challenges for central banks. Improved language models enhance the analysis of central bank communication, while increased cyber risks highlight the need for robust regulations. Moreover, AI impacts labour markets and macroeconomic conditions, requiring central banks to adapt their tools and strategies.
Conclusion
This report underscores the transformative potential of AI and big holdings data in central banking. Demand systems enhanced by AI enable better policy design, market understanding, and stress testing. To maximise these benefits, central banks should advocate for more comprehensive holdings data to refine models and strengthen financial resilience.