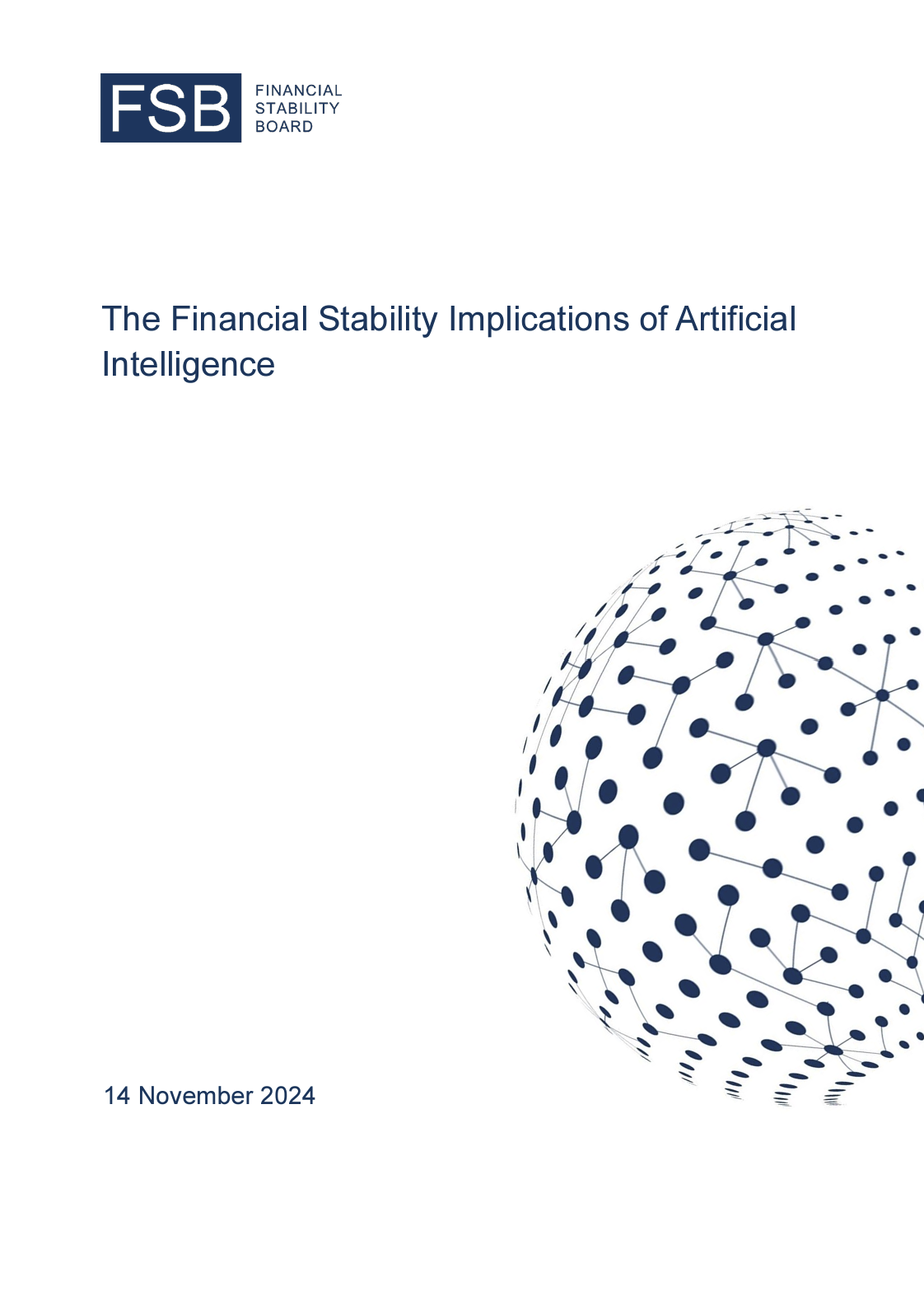
The financial stability implications of artificial intelligence
The report discusses the rapid adoption and integration of artificial intelligence (AI) in the financial sector, driven by advancements in technology and increasing operational efficiency. Key risks include dependencies on third-party providers, market correlations, and cyber vulnerabilities. Generative AI’s accessibility could amplify systemic risks, necessitating enhanced regulatory frameworks, vigilant monitoring, and robust governance to ensure financial stability amid evolving AI technologies.
Please login or join for free to read more.
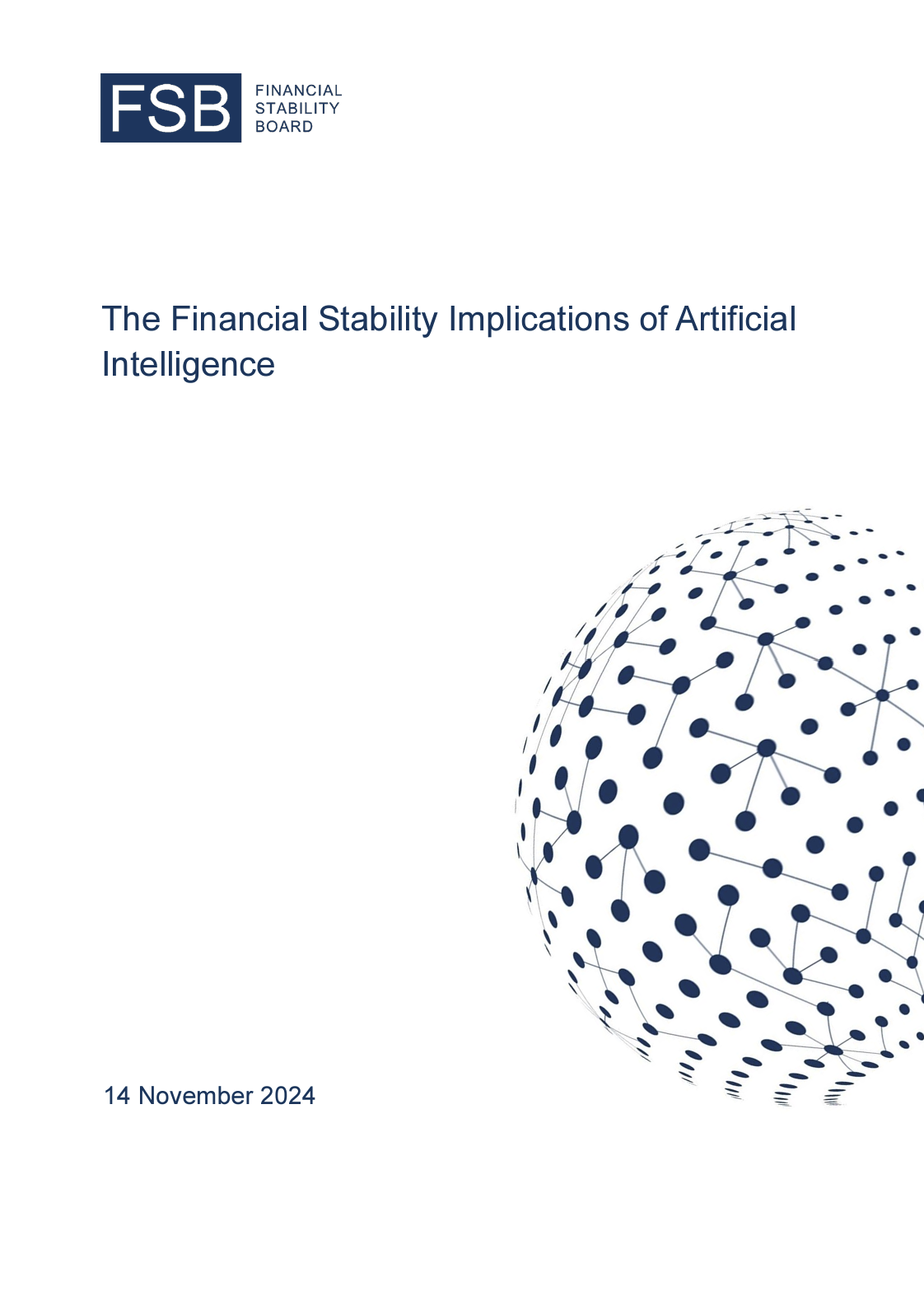
OVERVIEW
Introduction
The report examines advancements in artificial intelligence (AI) since 2017, focusing on its growing adoption, diverse use cases, and implications for financial stability. AI tools, particularly generative AI (GenAI) and large language models (LLMs), are reshaping financial services. However, rapid innovation and limited data on AI use create challenges for monitoring and mitigating associated risks.
Developments in AI since 2017
Technological advances, including deep learning, transformer architecture, and improved graphics processing units (GPUs), have accelerated AI adoption. The emergence of GenAI and LLMs, such as those leveraging transformer architecture, has transformed natural language processing (NLP). Financial institutions (FIs) now use unstructured data, including text and images, for training models.
Cloud computing and pre-trained models are key drivers of adoption, enabling firms to access cutting-edge AI tools without requiring extensive in-house expertise. Open-source models, which accounted for 66% of foundation models in 2023 (up from 33% in 2021), are expanding access but also introducing new risks, such as increased cyber vulnerabilities. By 2027, AI-related investment in finance is projected to rise from $166 billion in 2023 to $400 billion. However, data limitations may constrain progress, with high-quality datasets potentially depleting by 2026.
Selected use cases
AI supports efficiency, regulatory compliance, and customer interactions. In customer-focused applications, AI aids credit underwriting, particularly for individuals with limited credit history, and enhances marketing strategies by tailoring campaigns. GenAI enables the creation of scalable digital content and advances chatbot interactions, fraud detection, and insurance pricing.
Operational use cases include risk management, code generation, and capital optimisation. GenAI’s coding tools address skill shortages, allowing smaller firms to deploy AI models effectively. Supervisory authorities use AI for oversight, stress testing, and summarising inspection reports. Regulatory compliance, particularly in anti-money laundering (AML) and fraud prevention, has benefited significantly from AI’s adoption.
Financial stability implications of AI
AI adoption introduces systemic risks. Third-party dependencies on highly concentrated markets for GPUs, cloud services, and pre-trained models pose operational vulnerabilities. Market correlations could increase as FIs use similar AI models and datasets, potentially amplifying market stress. Automation in financial systems may exacerbate these vulnerabilities during periods of volatility.
Cybersecurity risks are heightened by GenAI, which lowers barriers for cybercriminals. Threat actors can leverage AI to execute phishing attacks, create synthetic identities, and compromise business emails. Governance issues, including opaque training data and model complexity, increase risks of errors and limit explainability during crises.
Key developments and monitoring challenges
The rapid pace of AI innovation complicates effective oversight. Financial regulators face skill gaps in data science and IT, impacting their ability to monitor vulnerabilities. Data exhaustion and the growing reliance on synthetic data introduce quality concerns. Additionally, smaller FIs may struggle to adopt AI, potentially widening resilience gaps.
Recommendations and policy implications
The report recommends addressing data gaps by conducting surveys, enhancing public disclosures, and engaging with AI developers and financial institutions. Regulatory frameworks should be assessed for adequacy, with specific focus on model risk, cybersecurity, and AI governance. Jurisdictions like the EU are implementing AI-specific regulations, such as the AI Act, to address transparency and ethical alignment. Enhanced regulatory and supervisory capacities, including the use of supervisory technology (SupTech), are crucial. International cooperation is necessary to harmonise standards and practices across sectors.
Conclusion
AI offers significant benefits in operational efficiency, regulatory compliance, and customer service. However, it amplifies systemic risks, including third-party dependencies, market correlations, and cybersecurity vulnerabilities. AI-driven changes in macroeconomic conditions, market competition, and energy consumption may introduce further complexities. Current regulatory frameworks address many risks, but gaps remain in monitoring, governance, and regulation. Continuous engagement, research, and adaptive policies are essential to ensure financial stability as AI adoption grows.