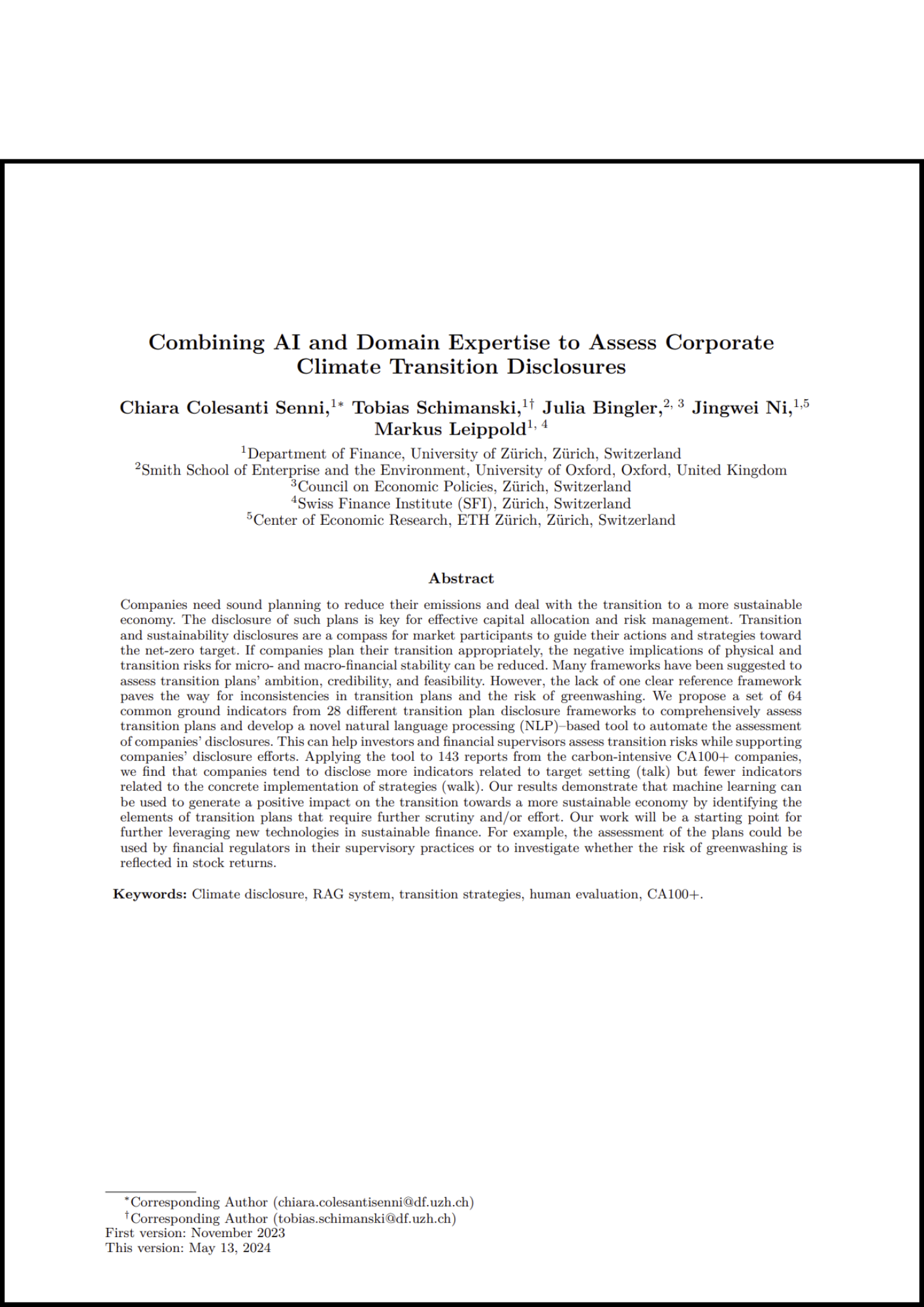
Combining AI and domain expertise to assess corporate climate transition disclosures
This paper introduces an assessment framework for corporate disclosures on climate transition strategies. It identifies 64 indicators from 28 frameworks, develops a natural language processing (NLP) tool to automate disclosure assessment, and analyses disclosures of carbon-intensive companies. The findings reveal that companies often prioritise reporting targets over concrete implementation actions.
Please login or join for free to read more.
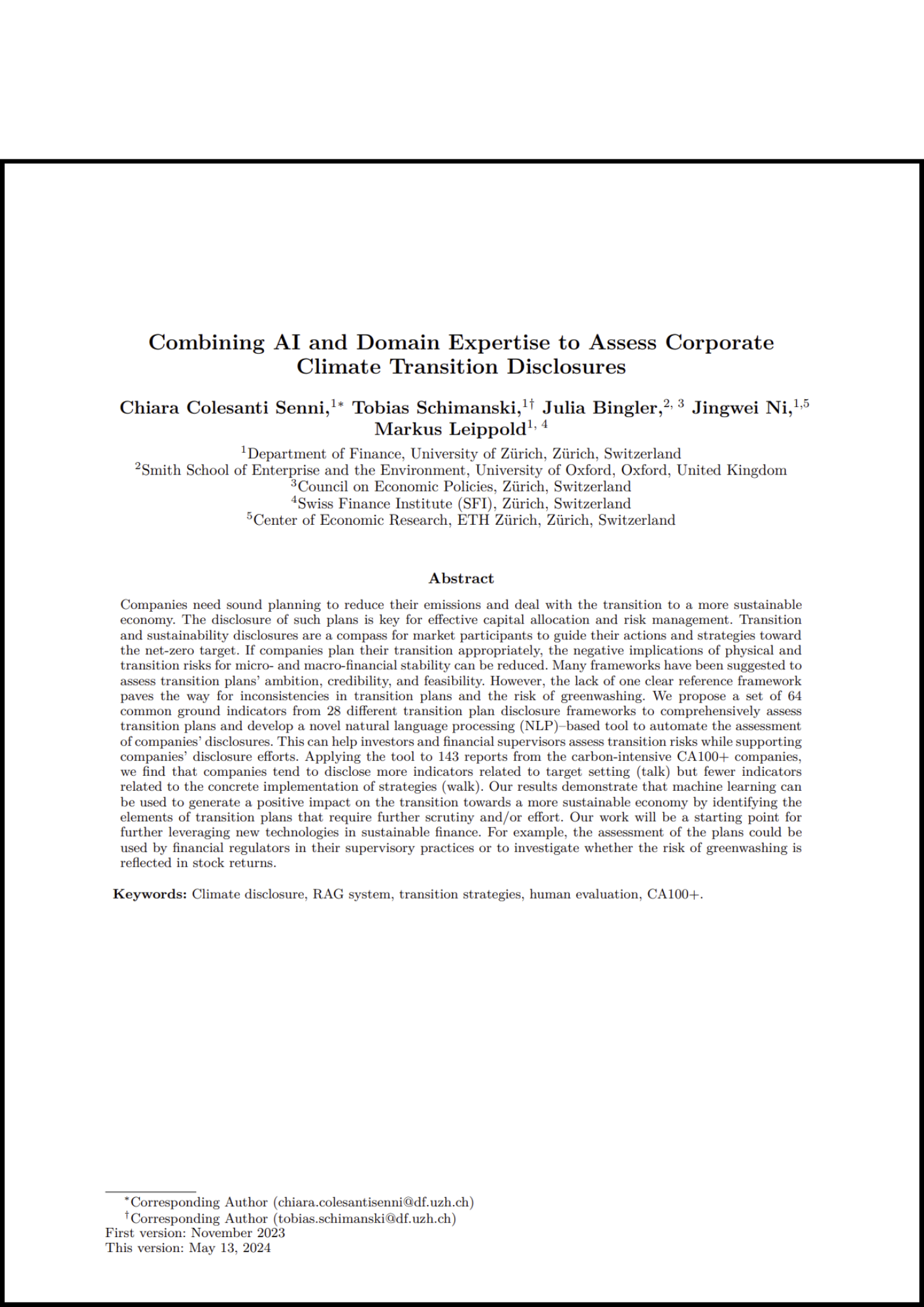
OVERVIEW
NLP model development
The report outlines the development of a natural language processing (NLP) tool designed to automate the analysis of corporate climate transition disclosures. This tool focuses on assessing a company’s alignment with various climate indicators, including targets for net-zero emissions and tracking of greenhouse gas (GHG) emissions across scopes 1, 2, and 3. The NLP tool leverages large language models (LLMs) and retrieval-augmented generation (RAG) techniques to process corporate reports and generate responses to predefined climate-related questions.
Human evaluation
A pilot study involving 26 institutions, including financial regulators and investors, was conducted to assess the tool’s performance. Participants evaluated the tool’s responses along several qualitative dimensions, including system quality, trustworthiness, and usage. A total of 396 indicator assessments were collected from 93 reports. Overall, 81% of participants found the tool’s summarisation of content accurate, while 12% noted partial satisfaction, and 6% were dissatisfied. Feedback revealed that, while the tool summarises content well, it sometimes omits critical information or lacks depth in its analysis. Additionally, the tool assists in identifying potential greenwashing by focusing on companies’ “talk” (target-setting) versus “walk” (strategy implementation).
CA100+ companies use case
The tool was applied to the disclosures of 143 companies from the CA100+ initiative, a group tracking high-emission firms globally. The average company disclosed 23 out of 64 climate indicators, with the best-performing companies disclosing up to 43 indicators. Approximately 35% of companies achieved a 40% disclosure rate. Sectoral discrepancies were evident, with companies in sectors like fossil fuels often lagging in key areas, especially regarding phasing out fossil fuel exploration and supply infrastructure. This sectoral variation suggests that while some companies disclose more indicators, the quality and comprehensiveness of their transition strategies vary significantly.
Most and least disclosed indicators
The most disclosed indicators included scope 1 GHG emissions and renewable energy strategies, while the least disclosed involved carbon offsetting practices and supply chain engagement on emissions. The analysis suggests that many companies excel in reporting short-term emissions data but lack comprehensive long-term strategies or transparent tracking of emissions reductions. For instance, only 43% of companies reported detailed renewable energy strategies, while a smaller percentage provided clear information on carbon offsets and long-term emission reductions. Additionally, few companies provided robust scenario analysis or sensitivity assessments to plan for varying climate outcomes.
Recommendations
The report suggests that the NLP tool can significantly reduce manual workload for stakeholders by automating parts of the disclosure assessment process. However, it also notes that the tool is not a substitute for human judgement and verification. Future improvements could focus on integrating more granular, sector-specific data and refining the tool to address criticisms regarding the omission of critical information or overinterpretation of data. It also highlights the importance of addressing greenwashing concerns by ensuring companies not only disclose future targets but also provide verifiable plans and actions to achieve those targets.
Conclusion
This study provides valuable insights into the effectiveness of an AI-driven approach to corporate climate transition disclosure analysis, with opportunities for enhancement. It offers an efficient method to assess disclosures, but greater sector-specific analysis and deeper scenario planning are needed to fully trust corporate transition strategies.